Apple has reached a new Milestone with its latest language model, ReALM, which claims to surpass the capabilities of OpenAI’s GPT-4 in the intricate task of reference resolution.
This advancement is a technical victory and a potential paradigm shift in how humans interact with technology. Reference resolution, the ability to understand to whom or what a conversation refers, has long been a stumbling block for AI, hindering seamless and intuitive interaction between humans and machines.
Apple’s achievement with ReALM, as detailed in their recent publication, suggests a future where technology can understand us with unprecedented clarity and precision.
This introduction sets the stage to explore the significance of ReALM, how it compares to the current gold standard set by GPT-4, and what this means for the future of artificial intelligence and its integration into our daily lives.
Overview of ReALM
ReALM, Apple’s latest foray into artificial intelligence, is a testament to the company’s dedication to pushing the boundaries of AI language models.
This model, designed with a keen focus on reference resolution, aims to bridge the gap between human communication and machine interpretation, making significant strides in understanding context and user intent in a way previously thought to be the exclusive domain of human cognition.
Apple’s ReALM is a concerted effort to tackle one of the most nuanced challenges in AI: the ability of a machine to understand references made in human language, such as “this,” “that,” “he,” or “she,” and to whom or what these pronouns refer in a given context.
While seemingly simple on the surface, this challenge requires deep contextual understanding and has implications for a wide range of applications, from enhancing conversational AI to improving user interaction with smart devices.
ReALM is engineered to excel in reference resolution across various contexts, distinguishing itself through its ability to process and interpret three types of entities:
Objects or information displayed on a user’s screen.
Elements that are part of an ongoing conversation or dialogue.
Aspects that may not be immediately visible or part of the active user interface are relevant to the user’s current context or environment, such as background applications or notifications.
This classification system enables ReALM to provide tailored responses based on a comprehensive understanding of the user’s current interaction context, setting a new standard for AI’s role in enhancing the user experience.
ReALM demonstrated its prowess in benchmark tests by outperforming GPT-4, a leading language model by OpenAI, particularly in tasks requiring nuanced reference resolution.
Apple’s research indicates that even the most minor model variant of ReALM achieves significant improvements over existing systems, with larger models showing even more substantial gains.
This performance is attributed to ReALM’s sophisticated understanding of context and ability to integrate and interpret multimodal inputs, such as text and images, for a more holistic understanding of user queries.
Integrating ReALM into Apple’s ecosystem could revolutionize user interaction with their devices, offering a more intuitive, natural, and efficient way to communicate with technology.
By understanding references more accurately, ReALM can enhance a wide array of applications, from Siri and search functions to accessibility features, making technology more accessible and user-friendly.
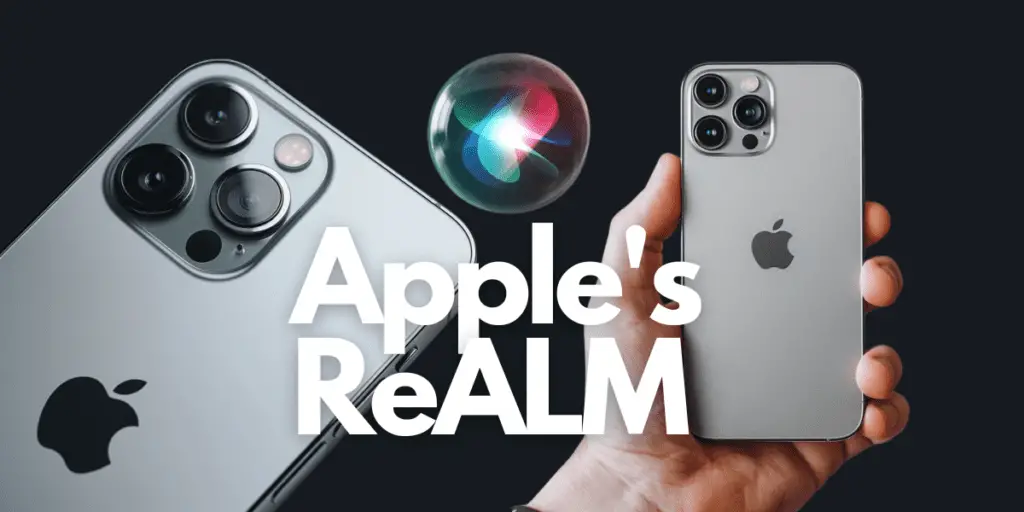
Reference Resolution Explained
Reference resolution is a fundamental aspect of human language understanding. It enables us to comprehend whom or what is being referred to in conversation or text.
This linguistic phenomenon is deceptively complex. It requires the listener or reader to contextualize and connect various pieces of information to determine the intended meaning behind pronouns like “he,” “she,” “it,” or phrases like “that one.”
This process often happens subconsciously for humans, drawing on our innate ability to integrate context, prior knowledge, and situational cues.
Mastering reference resolution is a formidable challenge for artificial intelligence, particularly language models like ReALM or GPT-4.
AI must navigate the nuances of language and context without the benefit of human intuition or the full spectrum of non-verbal cues that people naturally use to understand references.
The difficulty lies in the AI’s ability to discern, for instance, to which “he” a speaker is referring in a conversation involving multiple males or what “that” refers to among several previously mentioned objects.
Making chatbots and virtual assistants more intuitive and capable of sustaining coherent, context-aware conversations.
Allowing users to interact with technology using natural language makes commands like “turn off the lights in the living room” easily understood by smart home devices.
Enabling more sophisticated tools for individuals with disabilities, where nuanced language comprehension can provide more personalized and practical support.
AI models tackle reference resolution using various linguistic, statistical, and machine-learning techniques.
These models are trained on vast datasets to recognize patterns and infer references based on context, word usage, and the structure of the conversation.
The effectiveness of these models can vary greatly, influenced by their training data, underlying algorithms, and the complexity of the language used.
Apple’s ReALM model represents a significant advancement in this area. It is specifically designed to excel at reference resolution across different contexts.
By integrating the understanding of onscreen, conversational, and background entities, ReALM aims to provide a more nuanced and accurate interpretation of references, significantly enhancing the AI’s ability to interact in a human-like manner.
Understanding and improving reference resolution in AI offers a pathway toward more natural and efficient human-computer interactions, marking a critical step in the evolution of AI technologies.
As models like ReALM become more adept at this task, we edge closer to a future where AI can seamlessly integrate into our daily lives, understanding and assisting us in ways that are currently unimaginable.
Analysis ReALM vs GPT-4
In the landscape of artificial intelligence and natural language processing, the comparative analysis between Apple’s ReALM and OpenAI’s GPT-4 sheds light on language models’ evolving capabilities, particularly in the nuanced task of reference resolution.
This comparison highlights the technological advancements Apple made and sets the stage for understanding the future trajectory of AI interactions.
ReALM is Apple’s innovative approach to enhancing AI’s understanding of context and reference. It’s explicitly designed to improve how AI systems resolve references made in conversation or text, such as pronouns or contextual phrases.
ReALM distinguishes itself by focusing on three types of entities: onscreen, conversational, and background entities. It aims for a comprehensive understanding that encompasses the entirety of a user’s interaction with their device.
GPT-4, developed by OpenAI, represents the latest in a series of generative pre-trained transformers known for their broad capabilities in generating human-like text based on the input they receive.
While not explicitly designed for reference resolution, GPT-4’s extensive training on diverse datasets enables it to perform impressively across a wide range of language tasks, including reference resolution, to a certain extent.
ReALM has been shown to outperform GPT-4 in tasks specifically designed to test reference resolution capabilities.
Apple’s claims are backed by data indicating that even its most miniature ReALM model achieves performance comparable to GPT-4, with larger models showing significant improvements.
GPT-4, while a versatile and powerful model, could have been optimised explicitly for reference resolution, especially in complex scenarios involving onscreen or background entities. Its performance, although strong, indicates the potential for specialized models like ReALM to offer improvements in targeted areas.
The potential applications of ReALM within Apple’s ecosystem suggest a strategic focus on improving user experience across iOS, macOS, and other platforms by making interactions with devices more intuitive and contextually aware.
This contrasts with GPT-4’s broader applicability across various domains, from content creation to coding assistance, reflecting the different objectives behind each model’s development.
The comparative success of ReALM over GPT-4 in reference resolution points to a growing trend in AI development: the emergence of specialized models designed to address specific challenges.
This trend underscores the importance of targeted improvements in AI’s understanding of human language, which could lead to more nuanced and sophisticated AI-human interactions.
The competition between ReALM and GPT-4 highlights AI research’s vibrant and dynamic nature, pushing the boundaries of what is possible in natural language processing and setting new benchmarks for future developments in the field.
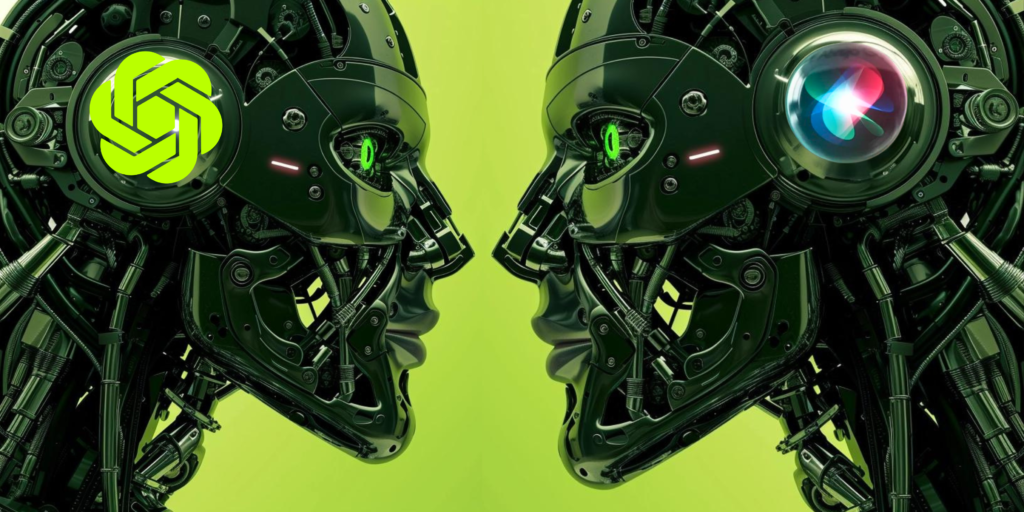
Integrating ReALM into Apple Products
The integration of ReALM into Apple’s product ecosystem could signify a transformative shift in how users interact with their devices. Advanced AI could leverage these interactions to make them more intuitive, contextual, and efficient.
Apple’s focus on enhancing ReALM’s capabilities in reference resolution tasks hints at a future where technology not only understands commands but also grasps their context within the user’s environment.
Integrating ReALM into Siri could revolutionize how users use voice commands to interact with their Apple devices.
Siri, powered by ReALM, could more accurately understand references, making interactions more natural and human-like. Referencing previous requests or mentioning onscreen content could become part of a fluid conversation with Siri, removing the need for repetitive explanations or clarifications.
Incorporating ReALM into the operating systems of iPhones, iPads, and Macs could lead to more brilliant context-aware features. Imagine typing a document or sending a message where the system understands references to earlier parts of the conversation or document, offering suggestions or corrections that are deeply contextual.
This could extend to more intelligent notifications, reminders, and search functions that understand the query and the context in which it’s made.
ReALM could significantly enhance accessibility features across Apple’s product line, offering users with disabilities more nuanced and effective ways to interact with their devices.
Reference resolution capabilities can make voice commands more flexible and forgiving, accommodating a broader range of speech patterns and making technology more accessible to everyone.
For Apple’s HomeKit ecosystem, ReALM could enable a more nuanced control over smart home devices through natural language commands that understand context and reference.
Users could control their smart home setups more naturally, referring to devices, rooms, or scenes with casual references that the system can interpret accurately.
CarPlay could benefit from ReALM in the automotive space by offering drivers a safer and more intuitive way to interact with their vehicles’ infotainment systems.
With enhanced reference resolution, drivers could make more natural and less distracting commands, such as referring to music, navigation points, or contacts in a conversational way.
As Apple continues to innovate, new products and services could be designed with ReALM’s capabilities in mind from the ground up, ensuring that future technologies are even more aligned with natural human communication patterns.
This could include virtual and augmented reality products, where reference resolution is critical for interacting with virtual objects or navigating augmented environments.
Integrating ReALM into Apple’s products promises to redefine the user experience, making technology more responsive, personal, and intuitive.
By advancing the understanding of context and references, Apple is poised to set new standards for AI interactions and further cement its role as a leader in innovation and user experience design.
Implications for the Future of AI
Apple’s development and integration of ReALM marks a significant milestone in the evolution of artificial intelligence, particularly in the domain of natural language processing.
This breakthrough showcases AI’s potential to understand and process human language with greater accuracy and sets a new direction for the future of AI development.
The implications of ReALM’s success in reference resolution are broad and far-reaching, extending beyond Apple’s ecosystem to influence the entire field of AI.
ReALM’s proficiency in understanding contextual references dramatically improves the quality of interaction between humans and AI.
This advancement means AI can participate in conversations that feel more natural and human-like, understanding references as a person would. It paves the way for AI systems to seamlessly integrate into daily life, assisting with tasks and providing information more intuitively.
With ReALM, AI has the potential to offer more personalized experiences to users by understanding the context of interactions and adapting responses accordingly.
This level of personalization can enhance user satisfaction and engagement across various applications, from virtual assistants to customer service bots.
Improved reference resolution capabilities can make technology more accessible to individuals with disabilities, offering them more independence and ease of use.
Integrating advanced reference resolution capabilities like those found in ReALM can revolutionize numerous industries.
AI could better understand patient descriptions of symptoms and medical histories in healthcare, improving diagnostic tools and patient care. In education, personalized learning experiences could be created based on the AI’s understanding of a student’s references to previous lessons or concepts.
The possibilities extend into every sector where natural language interaction with AI can enhance efficiency, accuracy, and user experience.
As AI systems like ReALM become more integrated into personal and professional spaces, ethical considerations and privacy concerns become increasingly significant.
The ability of AI to understand context and references raises questions about data collection, consent, and how this information is used. Ensuring that advancements in AI continue to respect user privacy and ethical guidelines is paramount for fostering trust and acceptance among users.
ReALM’s achievements in reference resolution challenge existing AI models to improve their understanding of human language, setting new benchmarks for what AI can achieve. This competition drives innovation in the field, encouraging the development of more sophisticated AI systems that can more proficiently tackle complex language tasks.
The implications of ReALM for the future of AI are both exciting and profound. As AI systems become better at understanding the nuances of human language and context, the potential for AI to augment human capabilities and transform society increases.
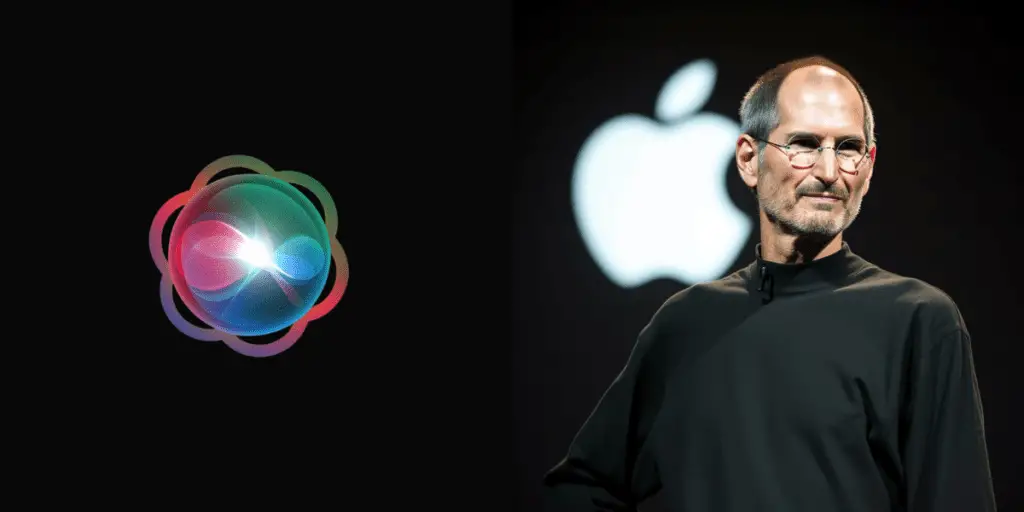
Final Thoughts
The advent of Apple’s ReALM and its touted superiority over OpenAI’s GPT-4 in reference resolution heralds a significant leap forward in artificial intelligence.
This development underscores the increasing sophistication of language models and their capacity to understand and process human language in a nuanced and contextually aware manner.
ReALM’s achievements in accurately resolving references within conversations and texts open up new possibilities for human-computer interaction, making these exchanges more intuitive, natural, and efficient.
Integrating ReALM into Apple’s suite of products and services promises to enhance user experiences across the board, from more responsive virtual assistants to more personalized interactions with devices and applications.
The implications of this advancement extend beyond the Apple ecosystem, setting new standards for AI development and application across industries.
As AI systems become more adept at understanding the subtleties of human language and context, we can anticipate a future where technology aligns more closely with human needs and communication styles.
The journey towards more advanced AI has its challenges. Ethical considerations, particularly regarding privacy and data security, remain at the forefront of the conversation.
Ensuring AI developments like ReALM adhere to stringent ethical standards is crucial in maintaining user trust and safeguarding personal information.
The comparison between ReALM and GPT-4 also highlights the dynamic nature of AI research and the ongoing quest for models that replicate human intelligence and complement and augment our capabilities.
As we stand on the brink of these technological advancements, it’s clear that AI has the potential to transform our relationship with technology, making it a more integrated and intuitive part of our daily lives.
ReALM’s introduction and potential integration into Apple’s product lineup represent a pivotal moment in AI. It signifies a step towards realizing the vision of creating technology that better understands us and can interact with us in more meaningful and contextually relevant ways.
As we move forward, the continuous evolution of AI promises to enhance our interaction with digital devices and redefine the boundaries of what technology can achieve.