Evolving landscape of artificial intelligence businesses increasingly use generative AI technologies to drive innovation, enhance efficiency, and offer novel solutions to complex problems.
The journey towards AI integration is fraught with challenges and limitations that developers and corporate users must navigate. At the forefront of addressing these challenges are Google and Anthropic, two leading entities in developing generative AI systems.
Their efforts were prominently featured during discussions at The Wall Street Journal CIO Network Summit held in Menlo Park, California.
Here, executives from Google and Anthropic candidly acknowledged the current limitations of their AI technologies, including the tendency of these systems to produce “hallucinations”—authoritative yet erroneous outputs.
As they refine these technologies, they also strive to balance the ambitious push for innovation with the pragmatic need to ensure reliability and accuracy in business applications.
This introduction sets the stage for a deeper exploration of the inherent challenges of generative AI, the strategies employed by Google and Anthropic to mitigate these issues, and the broader implications for businesses eager to harness the power of AI amidst a landscape of uncertainty and rapid technological change.
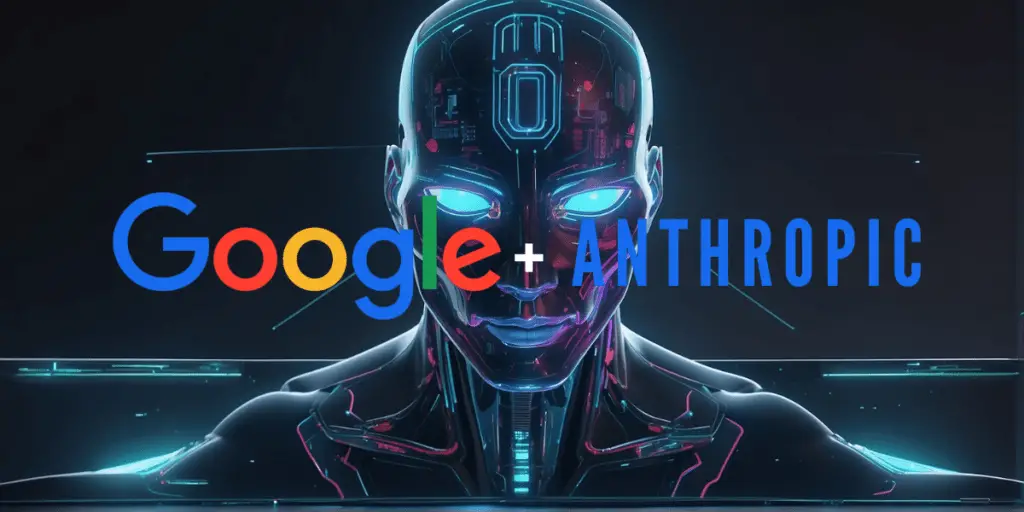
The Reality of AI Limitations
The burgeoning field of generative artificial intelligence has introduced a paradigm shift in how businesses approach problem-solving, innovation, and customer engagement. Despite the promising advantages, the reality of AI’s limitations has become a significant concern for developers and users alike.
A critical challenge that has emerged is the phenomenon known as “hallucinations,” where AI systems, in their attempt to generate responses, produce information that is not just inaccurate but often misleading or completely fabricated.
This issue was highlighted at The Wall Street Journal CIO Network Summit in Menlo Park, California, where leaders from Google and Anthropic, two trailblazers in the AI domain, openly discussed the limitations of their technologies.
The acknowledgment of hallucinations underscores broader challenges facing AI development and deployment. These include not only the generation of false information but also the efficiency of training these models, the removal of copyrighted or sensitive data from their training datasets, and the overarching difficulty in ensuring the reliability and veracity of AI-generated content.
These challenges are not trivial. They directly impact the trustworthiness of AI applications in business settings, where accuracy and reliability are paramount, especially in highly regulated sectors or dealing with sensitive information.
The difficulty in removing copyrighted or sensitive data from training materials further complicates the situation, as it raises legal and ethical concerns regarding using proprietary information without consent.
The efficiency of training AI models is another critical concern. The process requires substantial computational resources and time, making it a costly endeavor that can limit the ability of smaller enterprises to leverage advanced AI technologies.
This issue is compounded by the fact that once an AI model is trained on a dataset, it is challenging, if not impossible, to “unlearn” specific data or biases it may have acquired, thereby perpetuating potential inaccuracies or ethical issues.
As Google and Anthropic continue to develop and refine their AI technologies, acknowledging these limitations is a crucial step toward addressing them. It also serves as a reminder of the complexities involved in creating AI systems that are powerful and innovative but also reliable and ethical.
For businesses looking to integrate AI into their operations, these challenges underscore the importance of proceeding cautiously, ensuring that their reliance on AI technologies is balanced with a keen awareness of their limitations.
Corporate Skepticism
As generative artificial intelligence technologies advance, they bring potential applications across various sectors. This technological promise is met with skepticism from the corporate world, particularly in industries that are highly regulated or deal with highly sensitive information.
The acknowledgment by leading AI developers, such as Google and Anthropic, of issues like AI “hallucinations” and the challenges in ensuring data accuracy and security has only heightened this caution.
The concern over the reliability and trustworthiness of AI-generated content is at the heart of corporate skepticism. The stakes are high for businesses, especially when making decisions based on AI recommendations or using AI to interact with customers.
The fear that AI systems might produce misleading or incorrect information—however inadvertently—poses a significant risk, potentially leading to financial loss, reputational damage, or regulatory penalties.
The Wall Street Journal CIO Network Summit in Menlo Park, California, vividly illustrated this cautious approach to AI adoption. Lawrence Fitzpatrick, the Chief Technology Officer of financial services company OneMain Financial, voiced a question that resonates with many corporate technology leaders.
How can businesses confidently deploy AI applications in domains where accuracy and compliance are non-negotiable. Fitzpatrick’s query encapsulates the dilemma many organizations face: the desire to leverage the benefits of AI against the imperative to mitigate its risks.
The response from AI developers suggests a recognition of these concerns and a commitment to addressing them. For example, Anthropic’s co-founder and chief science officer, Jared Kaplan, highlighted ongoing efforts to reduce AI “hallucinations” by training models to acknowledge when they lack sufficient information to provide an answer.
Yet, this approach introduces another challenge: making AI models so cautious that they lose their utility, a balance that Kaplan likened to the difference between a non-hallucinating rock and a useful AI system.
Despite these assurances, the transition to AI-powered operations remains fraught with business challenges. The difficulty lies in the technical aspects of making AI systems more reliable and transparent and in the broader issues of data privacy, copyright infringement, and ethical use of AI.
As Google and Anthropic navigate these waters, the pace of AI adoption is likely to reflect a careful balancing act—eager to tap into the potential of AI but wary of moving too fast and risking too much.
The corporate world’s cautious adoption of AI underscores a critical phase in the technology’s evolution a move from unbridled enthusiasm for its potential to a more measured, risk-aware integration into business practices.
As AI technologies mature and developers like Google and Anthropic work to address their shortcomings, the hope is that corporate skepticism will give way to confidence.
Reaching this point will require technological advancements and clear regulatory frameworks, ethical guidelines, and robust mechanisms for ensuring the accuracy and safety of AI applications in the business context.
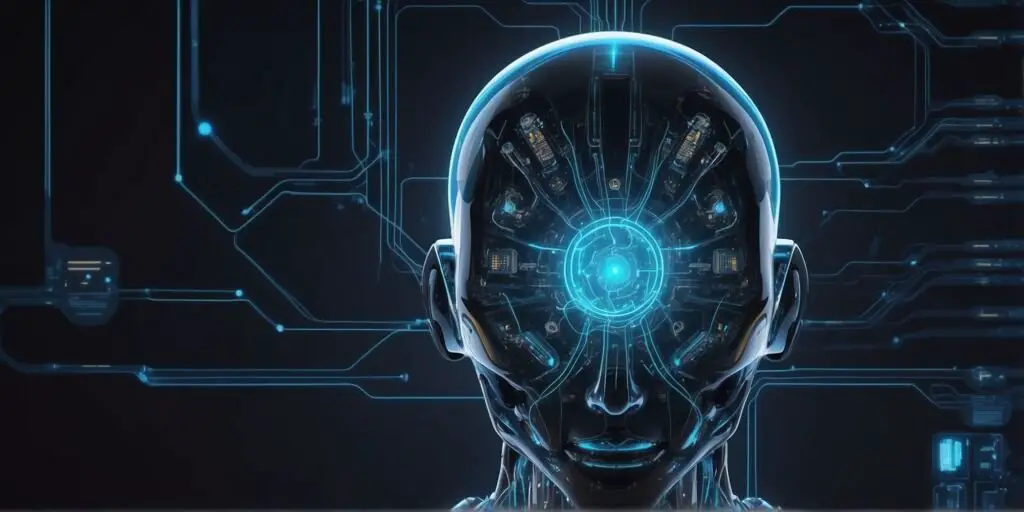
Strategies to Mitigate AI Shortcomings
One of the primary challenges with generative AI is its tendency to produce hallucinations or convincingly wrong outputs. To combat this, Google and Anthropic is focusing on training models to recognize their knowledge’s limits and respond with “I don’t know” when appropriate.
This approach aims to cultivate a more cautious AI that prioritizes accuracy over speculation. By building datasets where the correct response is uncertainty, Anthropic hopes to reduce the frequency of hallucinations, thus making AI systems more reliable and trustworthy.
There’s a delicate balance between reducing errors and maintaining the utility of AI systems. As Jared Kaplan, co-founder and chief science officer of Anthropic, pointed out, an AI model trained never to hallucinate might become overly cautious, limiting its usefulness.
Kaplan’s analogy that “a rock doesn’t hallucinate, but it isn’t very useful” underscores the challenge of ensuring AI systems are both accurate and helpful.
The goal is to develop AI to navigate the fine line between caution and functionality, providing valuable insights without compromising reliability.
Google and Anthropic approach to mitigating AI shortcomings involves enhancing the transparency of AI-generated content. Eli Collins, vice president of product management at Google DeepMind, emphasized the importance of enabling users to verify the sources of information provided by AI systems.
This strategy is particularly relevant in light of concerns about the provenance of model training data and copyright issues. By making it easier for users to identify and check the sources behind AI responses, Google aims to build trust and foster a more critical engagement with AI outputs.
Both Google and Anthropic also address legal and ethical considerations, especially regarding using copyrighted or sensitive data in AI training.
The challenge of removing specific pieces of content from trained models highlights the need for more sophisticated data handling and model training techniques.
As Google and Anthropic navigate lawsuits and public scrutiny, their efforts to develop more ethical AI practices are crucial for ensuring that AI technologies can be deployed responsibly and sustainably.
As AI technologies continue to evolve, the strategies employed by Google and Anthropic to mitigate their shortcomings will play a critical role in shaping the future of AI in business and beyond.
By reducing hallucinations, balancing caution with utility, enhancing source transparency, and addressing legal and ethical issues, these companies are laying the groundwork for more reliable, trustworthy, and ethical AI systems.
Google and Anthropic progress, it will be important for the broader AI community to learn from these experiences and to continue refining and developing new approaches to overcome the challenges of generative AI.
Google and Anthropic’s Solutions
Google’s strategic partnership with Anthropic underscores the tech giant’s belief in the potential of generative AI to revolutionize various sectors. In a significant move, Google agreed to increase its investment in Anthropic to $2 billion.
This investment is a testament to Google’s commitment to advancing AI technology and its confidence in Anthropic’s approach to developing safer and more reliable AI systems.
The collaboration between Google and Anthropic is focused on research and development initiatives aimed at enhancing AI models’ accuracy and efficiency, addressing data privacy challenges, and ensuring AI’s ethical use.
A critical aspect of Google and Anthropic’s efforts to improve AI technology involves increasing the transparency of AI-generated outputs. Eli Collins, vice president of product management at Google DeepMind, emphasized the importance of enabling users to verify the sources of information that AI systems provide.
This approach is crucial in building user trust, particularly in light of concerns regarding the provenance of training data and copyright issues. Google aims to foster a more informed and critical engagement with AI technologies by making it easier for users to identify the sources behind AI responses.
Google and Anthropic are also focused on addressing the hardware limitations that currently pose barriers to building more powerful AI models.
The availability, capacity, and cost of AI chips, essential for training complex models, are significant challenges. Jared Kaplan, co-founder and chief science officer of Anthropic, highlighted the need for efficient computing sources to overcome these hurdles.
Google has been investing in research developments, including its in-house chips, called Tensor Processing Units. These TPUs are deployed in Google’s data centers, offering a more efficient and cost-effective solution for AI model training.
The collaboration between Google and Anthropic is yielding advancements in the efficiency and cost-effectiveness of AI models. Google’s latest iteration of its Gemini model is reportedly more efficient and cheaper to build than previous versions.
This improvement is crucial for scaling AI applications and making them more accessible to a broader range of businesses and industries.
The partnership between Google and Anthropic represents a forward-looking approach to addressing the complex challenges of generative AI.
By combining their resources, expertise, and innovative strategies, these companies are not only working to enhance the performance and reliability of AI technologies. Still, they are also setting new standards for the ethical use of AI.
As these efforts continue to evolve, they promise to shape the future landscape of AI technology, making it more transparent, trustworthy, and beneficial for businesses and society.
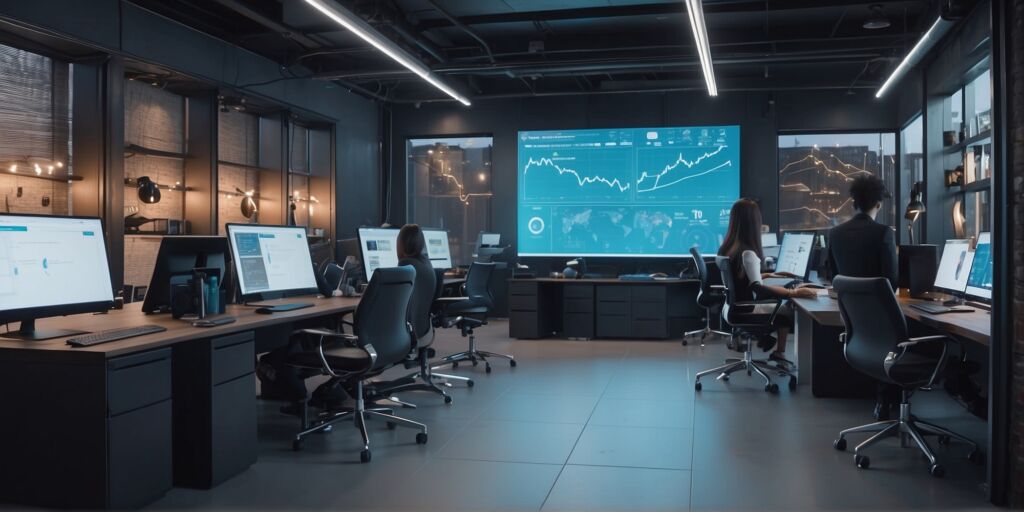
Legal and Ethical Considerations
One of AI developers’ most pressing legal challenges involves using copyrighted material in training AI models. A notable instance of this issue came to light in a lawsuit the New York Times filed against Microsoft and OpenAI.
The suit claimed that these companies utilized the Times’ content without permission to train their AI products, including the chatbot ChatGPT. This case highlights the broader problem of AI systems being trained on vast amounts of data sourced from the internet, where copyright ownership is often unclear or ignored.
The legal implications of using copyrighted content without authorization pose significant risks to AI developers, potentially leading to costly litigation and the need for reevaluating training datasets.
Another critical concern is the presence of sensitive or proprietary information in the data used to train AI models. Once an AI system is trained on certain data, removing specific pieces of content from its knowledge base is not straightforward.
This limitation raises questions about the control companies have over their proprietary information and the potential for AI to inadvertently reveal or misuse sensitive data. The risk of exposing trade secrets or confidential information through AI interactions necessitates robust data governance and privacy measures for businesses.
Beyond legal concerns, the ethical use of AI encompasses a wide range of issues, including bias, fairness, accountability, and transparency. AI models can perpetuate or even amplify biases in their training data, leading to unfair or discriminatory outcomes.
Ensuring that AI systems operate ethically requires careful consideration of the data they are trained on, the purposes for which they are deployed, and the potential impacts on individuals and society.
Developers like Google and Anthropic are increasingly focused on creating AI that is powerful, efficient, responsible, and aligned with ethical standards. AI developers are implementing various strategies to navigate these legal and ethical challenges.
These include developing techniques to identify and exclude copyrighted or sensitive information from training data, enhancing the transparency of AI models to allow users to trace the origins of AI-generated content, and adopting principles of ethical AI development that emphasize fairness, accountability, and transparency.
There is a growing call for regulatory frameworks to guide AI technologies’ ethical development and deployment, ensuring they contribute positively to society while respecting legal norms and individual rights.
The legal and ethical considerations surrounding generative AI are complex and evolving. As AI technologies advance, addressing these concerns will require ongoing efforts from developers, regulators, and the broader community to ensure that AI serves the public good while respecting copyright laws, data privacy, and ethical principles.
Technological Advancements
A fundamental challenge in advancing AI technology is the limitation imposed by current hardware. Training sophisticated AI models requires immense computational power, which can be costly and energy-intensive.
Google and Anthropic, among others, are investing in developing more efficient AI chips, such as Google’s Tensor Processing Units. These chips are designed to accelerate AI computations, making it feasible to train more complex models more efficiently.
Such hardware advancements are crucial for scaling AI applications and making them accessible to a broader range of users and businesses.
The efficiency and cost-effectiveness of AI models are critical considerations for their widespread adoption. Recent developments have made Google’s Gemini model more efficient and cheaper to build than its predecessors.
This trend towards more resource-efficient models is vital for democratizing AI technologies, allowing smaller entities to leverage advanced AI capabilities without prohibitive costs. As models become more efficient, they open up new possibilities for real-time applications and more sophisticated data analysis tasks.
As AI technologies become more embedded in daily life, the importance of ethical AI and transparency grows. Efforts to make AI models more understandable and accountable, such as enabling users to verify the sources of information AI systems provide, are gaining momentum.
This focus on transparency is crucial for building trust between AI systems and their users, particularly in sensitive applications such as healthcare, finance, and legal services.
Addressing ethical considerations such as bias, fairness, and the potential for misuse is becoming a central aspect of AI development, with companies adopting principles and guidelines to steer their AI practices.
The rapid advancement of AI technologies is outpacing existing legal and regulatory frameworks, leading to calls for updated laws and guidelines that address the unique challenges posed by AI.
Issues such as copyright infringement, data privacy, and the ethical use of AI are complex and multifaceted, requiring a collaborative effort between policymakers, technologists, and legal experts to resolve.
Developing comprehensive frameworks that support innovation while protecting individual rights and promoting ethical standards will be crucial for the future of AI.
Looking forward, AI technology’s trajectory is marked by excitement and caution. The potential for AI to transform industries, enhance productivity, and solve complex problems is immense.
Realizing this potential requires technological innovation and a commitment to ethical principles and societal values. As AI technologies continue to evolve, the focus will likely shift towards creating systems that are intelligent, efficient, responsible, transparent, and aligned with human interests.
The future of AI holds the promise of more personalized and intelligent services, breakthroughs in understanding complex data, and solutions to pressing global challenges.
Achieving these outcomes will depend on our ability to navigate the ethical, legal, and technical hurdles, ensuring that AI technologies are developed and used to benefit society.
Final Thoughts
The journey of generative artificial intelligence from a nascent technology to a pivotal force in modern business and society encapsulates its immense potential and its significant challenges.
As companies like Google and Anthropic push the boundaries of what AI can achieve, they also underscore the importance of addressing the technology’s limitations, ethical considerations, and legal implications.
The discussions and developments highlighted at events like The Wall Street Journal CIO Network Summit in Menlo Park, California, reveal a tech industry keenly aware of the need for responsible innovation.
The reality of AI’s limitations, particularly issues like “hallucinations” or generating misleading information, has prompted a concerted effort to enhance these technologies’ accuracy, transparency, and ethical use.
Strategies aimed at mitigating these shortcomings, including refining AI’s ability to recognize the limits of its knowledge and improving the transparency of AI-generated outputs, are critical steps forward.
Equally important are the investments in hardware and software that drive the efficiency and cost-effectiveness of AI models, making these technologies more accessible and practical for a broader range of applications.
Legal and ethical considerations remain at the forefront of the AI dialogue, challenging developers, users, and regulators to navigate a complex landscape of copyright issues, data privacy, and ethical usage.
Developing comprehensive legal and regulatory frameworks to keep pace with technological innovation ensures that AI serves the public good while respecting individual rights and societal values.
Looking to the future, the path of AI technology is one of both promise and caution. The potential for AI to revolutionize industries, enhance human capabilities, and address global challenges is immense.
Realizing this potential will require technological innovation and a steadfast commitment to ethical principles, societal engagement, and regulatory oversight. The ongoing efforts by leaders in the field to address the challenges and opportunities of AI reflect a broader understanding that the future of technology must be guided by a commitment to benefit humanity as a whole.
As we stand on the brink of what could be a new era in human history, shaped by the capabilities of generative AI, it is clear that the journey ahead will be as much about the values we uphold as the technologies we develop.
The balance between innovation and responsibility, between ambition and caution, will define the legacy of AI in the decades to come.