OpenAI introduced Sora, an artificial intelligence model that has captivated the tech world with its ability to generate high-definition videos from simple text prompts.
OpenAI Sora Diffusion marks a significant leap forward from previous models like Runway’s Gen-2 and Google’s Lumiere, introducing a new era where AI could potentially reshape the traditional filmmaking landscape.
Sora, powered by an advanced technology known as the Diffusion Transform, combines the robust capabilities of transformers and diffusion models. It may be going to set a new standard for video generation.
Sora Diffusion
The technological backbone of OpenAI’s Sora is rooted in two pivotal AI architectures: transformers and diffusion models.
Both have played a crucial role in redefining the landscape of artificial intelligence applications, especially in handling complex data types like text and images.
Below, we explore these core technologies and their integration in the form of Diffusion Transformers, which powers Sora. Transformers are a class of deep learning models that have revolutionized how AI systems process sequential data.
Initially designed to handle natural language processing tasks, transformers work by focusing on different parts of data simultaneously, enabling more dynamic and contextually aware interpretations.
This architecture is particularly adept at understanding and generating text, making it a cornerstone in developing large language models. Diffusion models represent a newer approach in machine learning, primarily used for generating high-quality images.
These models function by gradually transforming noise into structured data through steps, effectively ‘learning’ the distribution of natural images.
This process involves starting with a random distribution and iteratively refining it towards a target distribution, a technique that has proven highly effective for image synthesis.
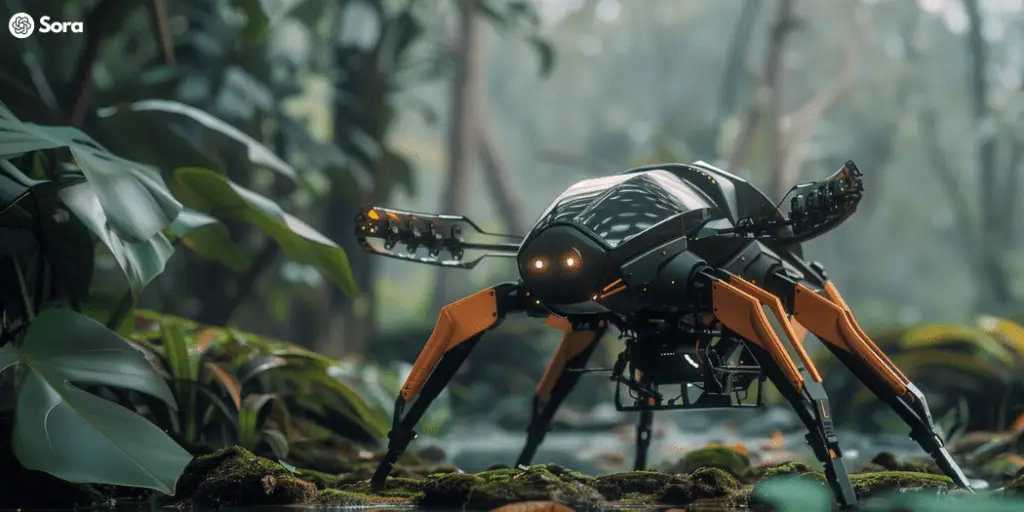
The Diffusion Transformer, or DiT, integrates the strengths of both transformers and diffusion models into a singular framework. Developed by innovators William Peebles and Saining XE, DiT replaces the traditional U-Net architecture commonly used in diffusion models with a transformer backbone.
This substitution aims to enhance the model’s performance by leveraging the transformer’s ability to handle complex patterns and dependencies in data.
DiT utilizes the diffusion process to handle video data, while the transformer architecture scales up the process, maintaining efficiency and quality even with significant inputs.
Fusing these technologies in Sora improves the fidelity and detail of generated videos. It exemplifies how combining different AI methodologies can lead to significant advancements in digital media production.
This integration signifies a significant step forward in AI-driven content creation, promising new entertainment, marketing, and beyond possibilities.
Functioning of Sora Using DiT
OpenAI’s Sora leverages the innovative Diffusion Transformer to transform text prompts into vivid, high-definition videos.
This process involves a sophisticated interplay of AI techniques that refine noisy input into clear and coherent visual outputs. Sora begins by dissecting a relevant video from its dataset into smaller segments or patches.
This is akin to breaking down a video into manageable puzzle pieces that are easier to analyze and reconstruct. Each video patch is then simplified or encoded into a less complex form.
This step helps reduce the data’s complexity, making it more manageable for the model to process. To introduce variability and creativity, random noise is added to these simplified patches.
This noise acts as a placeholder for potential changes and enhancements that might be applied to the video. The text prompt is converted into a numerical format that the model can understand.
This conditioned data is then integrated with the noisy video patches, aligning the video content with the thematic elements of the prompt. The model employs an attention mechanism to determine which parts of the video are most relevant to the prompt.
It assesses the importance of different elements within the patches, focusing on areas that are likely to contribute most significantly to the final output.
The model uses the transformer’s capabilities to iteratively refine each video patch, enhancing details and coherence. DiT progressively reduces the noise during this stage, clarifying and enhancing the video based on the input prompt and the initial noisy data.
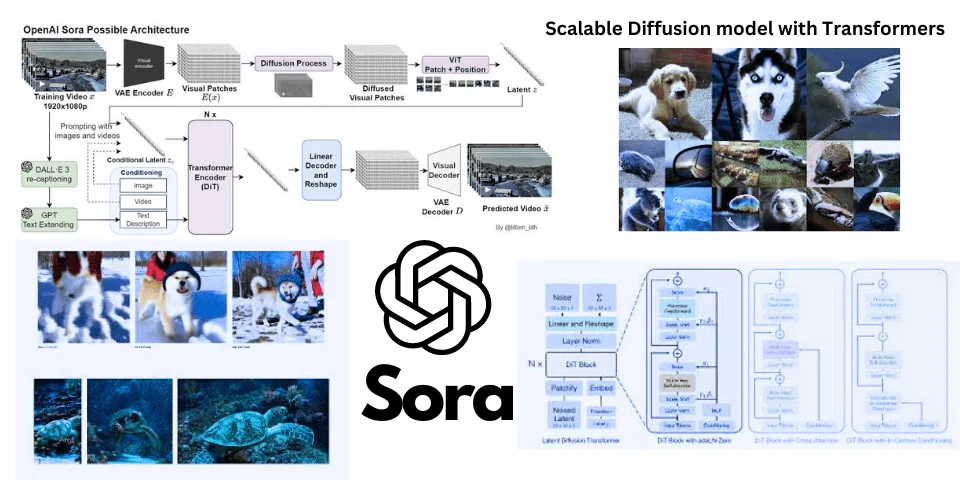
Once all patches have been individually refined, they are reassembled to form a complete video. This step involves stitching the patches together in a way that maintains continuity and flow, ensuring that the video appears seamless.
The final stage involves removing any remaining traces of noise and polishing the video to enhance its quality and clarity. This results in a crisp, clear video that accurately reflects the provided text prompt.
Through these stages, DiT enables Sora to understand and interpret text prompts but also to creatively transform these prompts into dynamic and detailed videos.
Video Generation Process
The video generation process employed by OpenAI’s Sora, powered by the Diffusion Transformer, is a complex yet fascinating sequence that transforms text prompts into detailed, high-definition videos.
Step 1 Prompt Interpretation and Video Patching
- A user inputs a text prompt, such as “Sora is sky”.
- Sora accesses its dataset to find a video related to the prompt. It then breaks this video into smaller segments, or patches, making the complex video data more manageable.
Step 2 Simplification and Noise Addition
- Each patch is converted into a simpler, summarized version. This process helps reduce the video data’s complexity, allowing the model to focus on essential elements.
- Random noise is introduced to these simplified patches. This noise is vital as it acts as a substrate to refine and enhance the video’s details.
Step 3 Conditioning with the Prompt
- The text prompt is encoded into numerical data that the model can process.
- This encoded prompt is integrated with the noisy patches, aligning the video data with the thematic elements of the prompt. This is a crucial step where the initial direction of the video content begins to take shape.
Step 4 Attention Pooling and Iterative Refinement
- The model employs an attention mechanism to assess which parts of the noisy video patches are most relevant to the prompt. This process ensures that significant areas are focused upon and enhanced.
- Using the transformer architecture, the model refines each patch in iterations, gradually reducing the noise and enhancing clarity and detail to better match the prompt.
Step 5 Reconstruction and Final Enhancement
- The refined patches are reassembled to form a coherent video. This step is critical as it involves stitching the patches together seamlessly, maintaining continuity and narrative flow.
- Finally, any residual noise is removed, and the video undergoes a polishing process to enhance its visual quality. This ensures that the video is coherent, aesthetically pleasing, and clear.
Final Output
- The completed video is presented as the final output, showcasing a clear, detailed, and contextually aligned video based on the original prompt.
This process exemplifies the power of combining advanced AI technologies like transformers and diffusion models in creative applications.
Sora’s ability to generate videos from text prompts opens up new possibilities in content creation, potentially transforming industries reliant on visual media.
Advantages of Diffusion Transformers
The Diffusion Transformer technology, which powers OpenAI’s Sora, represents a significant advancement in AI-driven content creation.
This integration of diffusion models and transformer architecture brings several compelling advantages that enhance the capabilities of AI systems in handling complex visual data.
DiT excels at generating high-quality images and videos by effectively managing the iterative refinement of noise into structured visual data. The use of transformers in this process allows for maintaining high fidelity and detail, even with complex visual content.
The transformer component of DiT enables better handling of details and nuances in images and videos. This results in clearer and more precise visual outputs, crucial for high-resolution media applications.
DiT’s transformer architecture allows it to effectively manage large and complex datasets without significantly decreasing performance. This scalability is vital for training on extensive video content and for applications that require dealing with vast amounts of visual data.
DiT optimizes computational resources by integrating transformers, which is crucial for processing video data that typically requires substantial computational power. This efficiency makes DiT suitable for more extensive and resource-intensive projects.
The flexible nature of DiT makes it adaptable to various types of content, from still images to dynamic videos. This versatility is essential for applications across different media types and industries.
The ability to condition the diffusion process with specific prompts allows for customizable outputs. This feature is particularly beneficial for creative industries where tailored visual content is necessary.
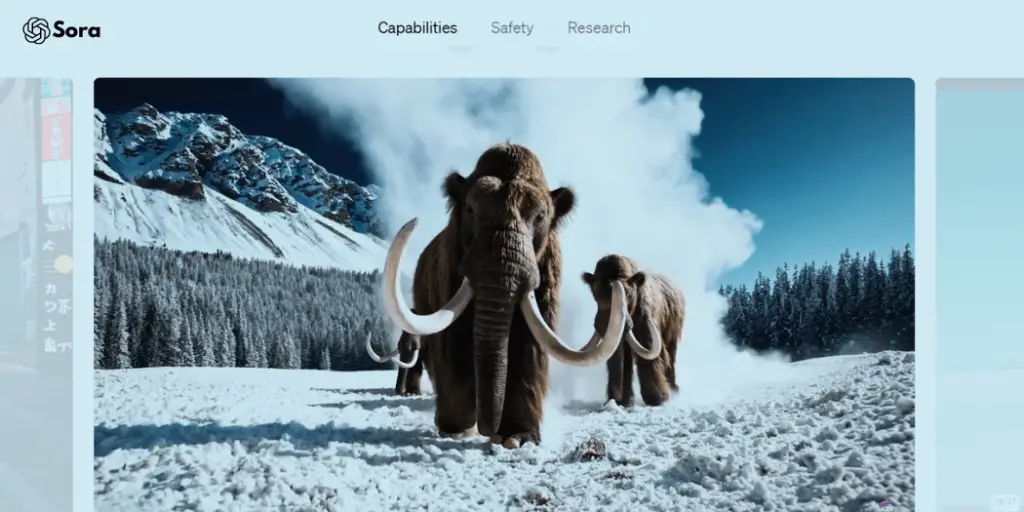
DiT refines visual data through iterative processes, continuously learning and improving the output based on the input and intermediate results. This continuous improvement helps in achieving better accuracy and quality over time.
The architecture supports a feedback mechanism where the system learns from its errors and refines its approach, leading to increasingly accurate predictions and enhancements in subsequent outputs.
The capabilities of DiT extend beyond traditional content creation, offering potential applications in areas such as virtual reality, augmented reality, and automated video editing.
DiT can transform the creative process in filmmaking, marketing, and other fields, reducing time and labor costs while increasing creative possibilities.
Final Thoughts
With the seamless integration of the capabilities of transformers and diffusion models, DiT provides a robust framework for generating high-definition videos from simple text prompts.
This breakthrough enhances the quality and efficiency of video production and opens up new possibilities for creativity and automation in various industries.
The potential of DiT to transform the filmmaking process is particularly notable.
With its ability to interpret and visualize complex prompts, Sora can potentially reduce the time and resources required for traditional video production, making high-quality video content more accessible to a broader range of creators.
Its scalability and flexibility make it an invaluable tool for applications ranging from entertainment and marketing to education and virtual reality. As AI advances, the implications of technologies like DiT extend beyond mere technical achievements.
They challenge our traditional notions of creativity and content creation, promising a future where AI partners with humans to explore new artistic horizons and communication forms.
This partnership could redefine industries, enhance creative expression, and, perhaps most importantly, unlock unprecedented opportunities for storytelling in an increasingly digital world.